In today's rapidly evolving AI landscape, large language models (LLM) are making waves across various industries. While these AI-driven models have undoubtedly transformed the way businesses interact with their audience, they do come with a few drawbacks – from generating misleading information to not being able to provide up-to-date information and raising transparency concerns.
This is where RAG comes into play!
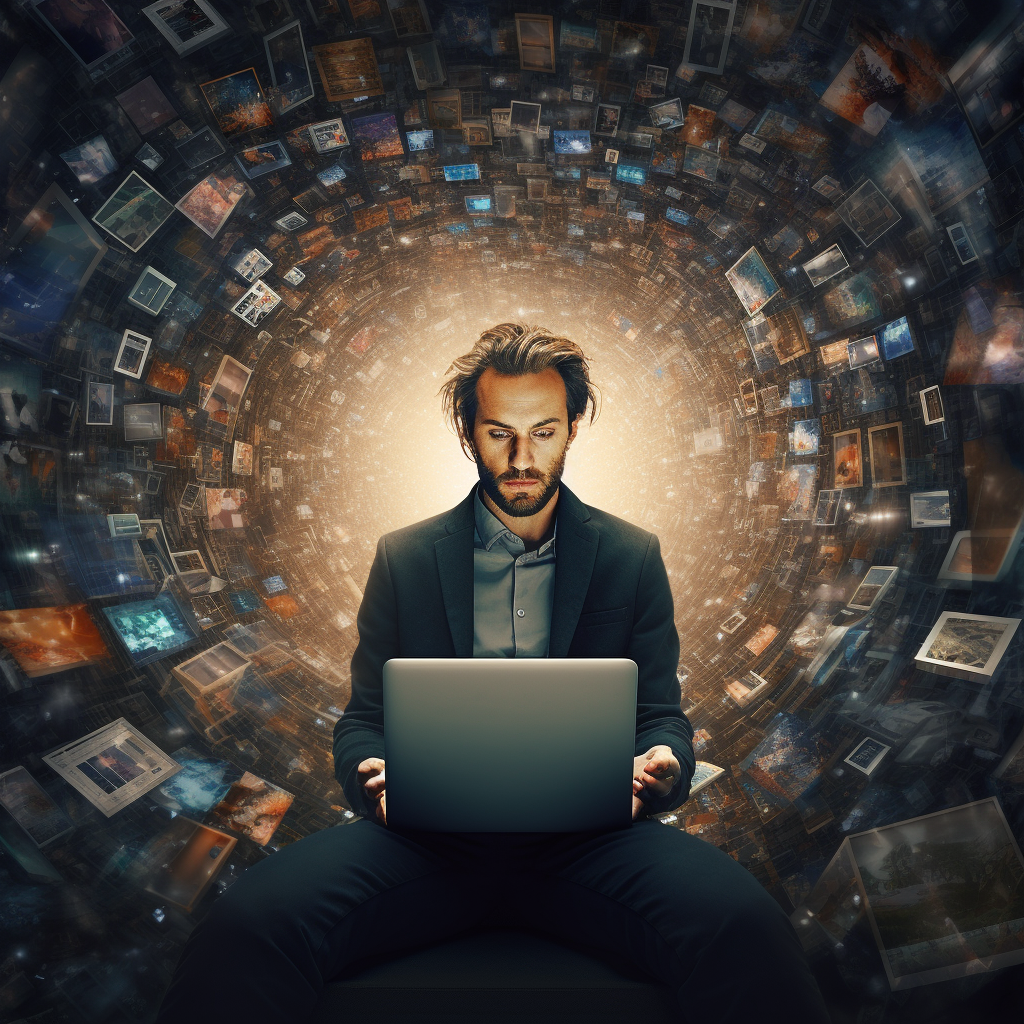
Retrieval-augmented generation (RAG) is a framework or architecture designed to address the limitations of large language models. By combining retrieval mechanisms with state-of-the-art pre-trained language model generation capabilities, RAG offers a more accurate and reliable solution for generating content and interacting with users. The enhanced performance of RAG can be attributed to its contextual understanding and grounded responses. This unique integration enables RAG to provide contextually relevant and accurate content, effectively addressing the common challenges faced by standalone language models.
In this article we will outline 10 reasons why you should consider using RAG as your go-to choice for implementing LLMs in your business. By the end of this article, you'll have a clear understanding of how RAG can benefit your business, reduce hallucinations, and provide credible, real-time access to domain-specific information. Let's dive in!
1. RAG Reduces Hallucination
Hallucination, or the generation of incorrect or misleading information, is a common challenge in large language models. The RAG framework significantly reduces this issue by combining the power of retrieval and generation. The RAG's retrieval component helps mitigate this problem by grounding generated content, ensuring the responses it generates are well-grounded and therefore minimising the chances of hallucination.
Example: In the financial services industry, providing accurate information on investment options is crucial because it directly impacts customers' purchasing decisions and financial well-being. RAG can help ensure that the information generated about stocks, bonds, or mutual funds is well-grounded in factual data, reducing the risk of misleading clients and potentially harmful financial decisions.
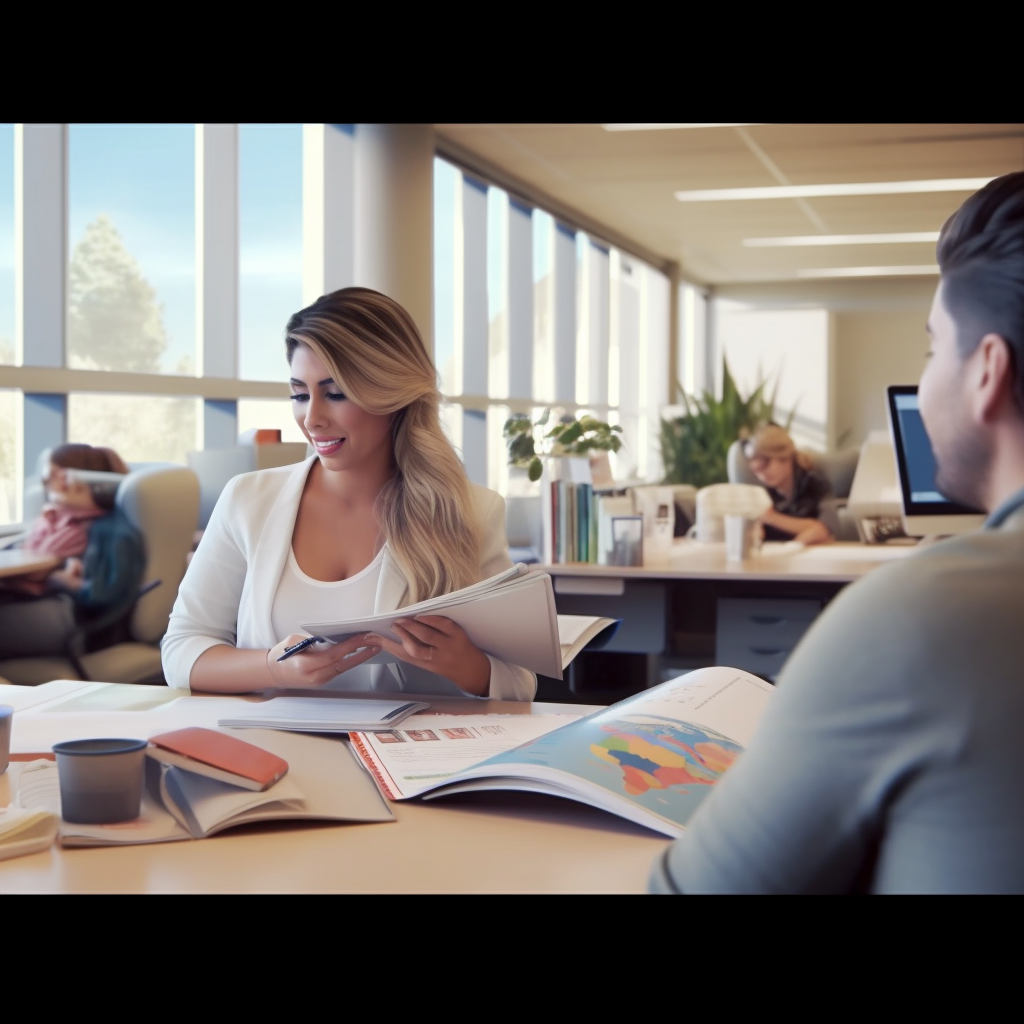.png)
2. Cost-Effective Alternative
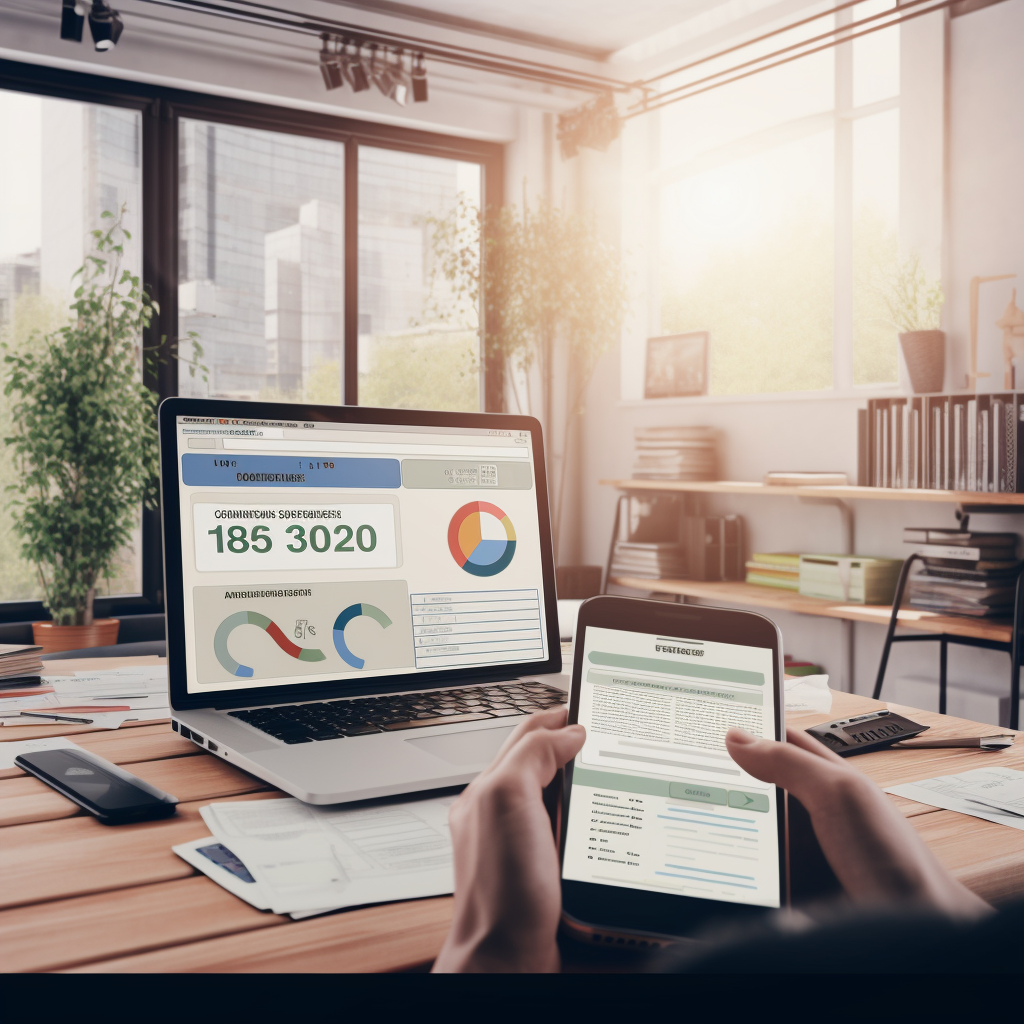.png)
Fine-tuning a pre-trained language model can be a resource-intensive process. However, RAG offers a cost-effective alternative by leveraging an existing model and augmenting it with retrieval mechanisms; this can save businesses both time and money while still achieving excellent results.
Example: Banks often need to assess the creditworthiness of potential borrowers. Fine-tuning pre-trained language models to analyse credit histories can be resource-intensive. RAG architecture offers a cost-effective alternative by retrieving relevant financial data and credit history information from existing databases, combining this with pre-trained language models to generate accurate credit assessments. This approach saves both time and money while still providing reliable results for banks.
3. Credible and Accurate Responses
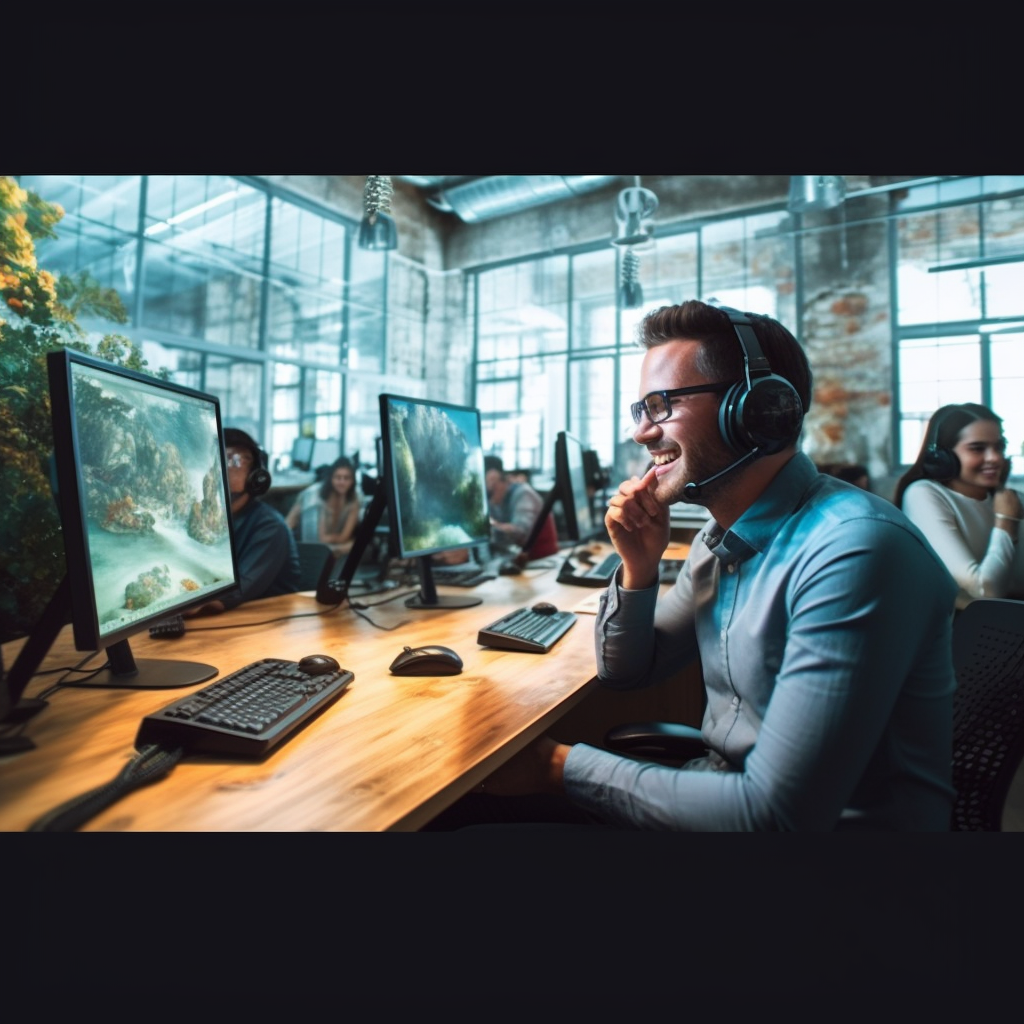.png)
One of the standout features of RAG is its ability to generate credible and accurate responses. RAG combines the power of retrieval mechanisms with advanced language model generation, resulting in more accurate and contextually relevant content. This means fewer errors and a better overall user experience. This is important for businesses looking to maintain their credibility.
Example: In customer support, providing accurate and helpful responses is essential for maintaining customer trust, as it demonstrates the company's commitment to providing reliable information and support. The RAG technique is able to do this very effectively by retrieving data from catalogues, policies, and past customer interactions to generate context-aware insights, ensuring that customers receive reliable information on product features, returns, and other inquiries.
4. Domain-Specific Information
RAG allows businesses to tap into domain-specific information. With its ability to access domain-specific knowledge bases, RAG can provide users with information tailored to their needs or requirements – something that just using LLMs may struggle with.
Example: In the legal industry, clients often require advice specific to their case or jurisdiction because different legal systems have unique rules and regulations, and understanding these nuances is crucial for effective legal representation. RAG can access domain-specific knowledge bases, such as local statutes and case law, to provide tailored information relevant to clients' legal needs.
5. Improved Transparency
One of the major concerns with AI models is their "black box" nature. RAG addresses this by being more transparent about the information sources it uses. When RAG generates a response, it can also cite the sources it drew from, enhancing transparency and instilling trust in your users.
Example: Transparency is critical when providing information on product sourcing, sustainability practices, or investment strategies because it helps build trust with customers and demonstrates the company's commitment to ethical practices. RAG can be used to retrieve information from trusted sources and generates insights, citing these sources to enhance transparency and consequently building trust.
6. Context-Aware Generation
One of the key advantages of RAG is its ability to generate context-aware responses by retrieving relevant data before crafting a response. This leads to clearer and more meaningful interactions with users, as the AI system understands the conversation's context, ultimately improving communication and user satisfaction.
Example: RAG's context-aware generation means that for example in the retail industry, marketing materials and product descriptions are tailored to individual customers, taking into account their preferences, purchase history, and demographics. This personalisation leads to better-targeted promotions and improved customer experiences.
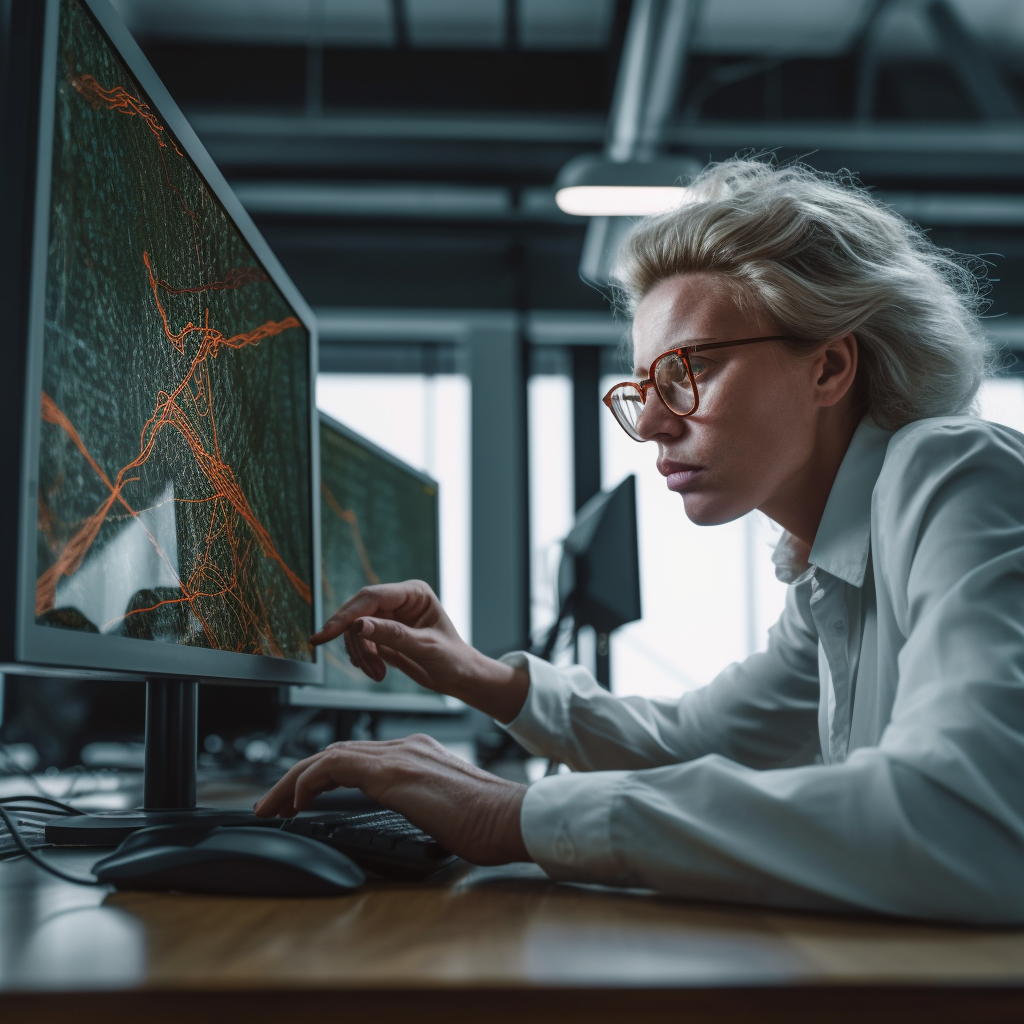.png)
7. Faster Processing
Another key benefits of RAG (Retrieval-Augmented Generation) is its ability to process vast amounts of data in real-time. This advantage empowers businesses to swiftly identify trends, patterns, and customer preferences, enabling them to make informed decisions and take action before their competitors.
Example: In the financial industry, real-time data analysis is vital for making informed investment decisions and take action before competitors, leading to improved competitiveness and customer satisfaction. RAG quickly processes large amounts of data, such as market trends and economic indicators, enabling financial institutions to identify opportunities and make timely decisions that benefit their clients.
8. Competitive Advantage
By implementing RAG, you gain a competitive edge. The technology allows you to stay ahead of the curve, ensuring that your business remains up-to-date, informed, and responsive to the ever-evolving demands of your industry.
Example: In the financial industry, staying ahead of market trends and regulatory changes is crucial for success. RAG retrieves and processes up-to-date market data and regulatory updates, allowing financial institutions to make informed decisions and stay ahead of their competitors.
9. Scalability
RAG offers scalability that caters to businesses of all sizes. Whether your business is a small startup or a large enterprise, RAG can accommodate your growing information needs. It has the ability to adapt and handle increased data and user interactions without compromising performance or accuracy. This makes RAG a future-proof solution for businesses looking to stay ahead in the AI-driven landscape and continue providing exceptional services to their customers.
10. Industry and Domain Agnostic
One of the standout benefits of using RAG is its incredible adaptability to virtually any industry or domain. This powerful framework isn't limited by specific sectors, making it a versatile solution for businesses across the board. Whether you're operating in finance, education, healthcare, e-commerce, or beyond, RAG's flexibility allows it to seamlessly integrate into any industries.
Wrapping up
In conclusion, Retrieval Augmented Generation (RAG) is a game-changer in the world of LLMs. With its unique ability to combine retrieval mechanisms and state-of-the-art generation capabilities, RAG ensures accurate, relevant, and context-aware content generation across various industries.
The ten key benefits of RAG, as highlighted in this article, make RAG an invaluable tool for businesses looking to stay ahead of the curve and continue providing exceptional services to their customers. So why not give RAG a try and see how it can benefit your business and help you stay ahead of the curve?
To get started with RAG, check out this YouTube video for more information.
Topics Covered :
Author
Gavita Regunath